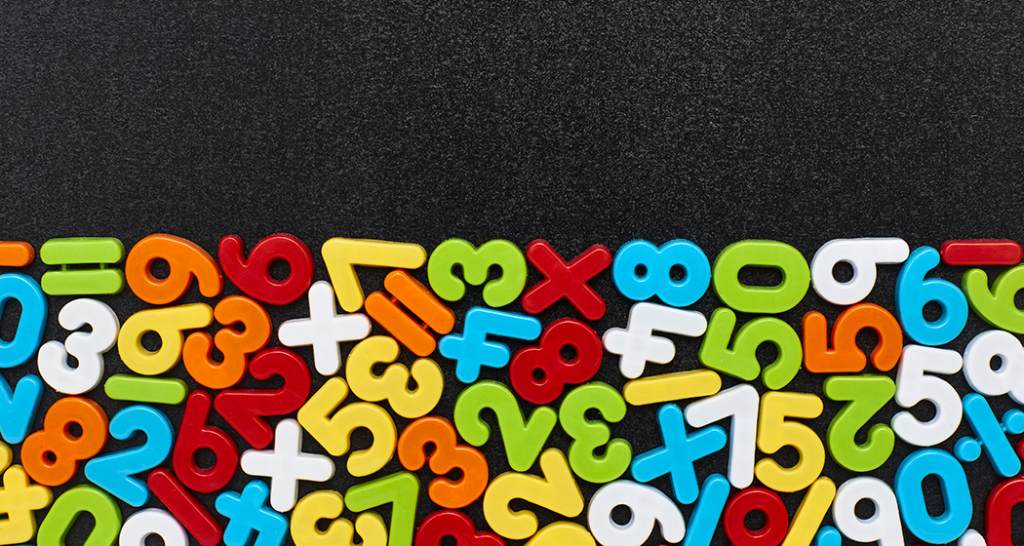
IESE Insight
In data we trust? A manager's guide to understanding quantitative methods
From pricing to forecasting models, and some useful managerial tips: what to expect from Quantitative Methods for Managers: A Practical Approach.
Is there a gender pay gap in your firm? Can you predict which borrowers will fail to pay back their loans? How might customers best be retained through promotions?
Data makes the world go around, and more and more managers are turning to academic methods to evaluate that data and make informed decisions for their companies. Yet for many, quantitative methods can be intimidating, especially when there's a focus on theory over practice.
Miguel Ángel Canela and Inés Alegre of IESE, with co-author Alberto Ibarra of IPADE, aim to redress this balance with a new textbook that provides a short introduction to the most important methods. Theory is reduced to the minimum in order to focus on real cases and practical applications.
The book is divided into four sections:
1: A warmup on summary statistics and probability distributions.
How can we describe the market behavior of Tata Motors' share price? The average return is a good starting point, but it is also critical to know its distribution and to understand the stock's volatility over time.
The authors use stock prices and other examples — such as the tweets generated during The EuroLeague Final Four competition — to explain the fundamentals of the mean, standard deviation and probability distributions. These basic concepts are often used in business decisions today, but could be used better.
2. The core of the course: regression analysis.
How does the price of an orange juice brand affect its market share? How might management react when competing brands change their prices? Modeling and analyzing multiple variables via various types of regression analyses occupies the core of the book. In fact, the authors assert: "Most business applications of quantitative methods are regression analyses, sometimes in disguise." While explaining the ins and outs of regression techniques with many practical cases, the authors also warn of their limits and common pitfalls.
3. Classification models.
Can you predict whether or not a customer will leave your company in favor of a competitor? Classification models are regression models where the predicted variable is categorical (e.g., gender or marital status). These models can help managers handle many complex situations in business — from detecting which emails are non-desired spam to predicting who will default on a loan.
4. Elementary methods of time series analysis.
Time series data (data ordered chronologically) are frequently used for sales forecasting. Different forecasting techniques are explained using quarterly and monthly data. Cases include forecasting beer sales as well as winter clothes and packaged meat.
With added Excel files and R code in the appendices, this book is intended for students and management practitioners. It doesn't seek to reinvent the wheel — indeed, all of its concepts are tried and true stalwarts in business studies — but it does aim to provide clear and focused advice to improve decision-making skills.