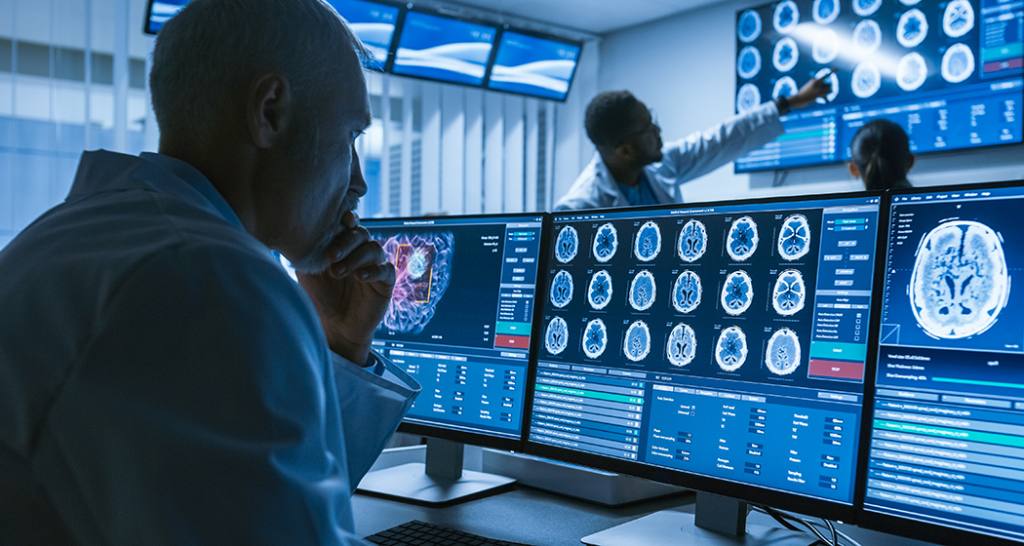
IESE Insight
When diagnosis is part of the service: Learning and treating efficiently
Is it just a fever or something more serious? When providing services that range from simple to complex, as in health care, not knowing what's required for each patient leads to wasted resources and inefficient staffing. So, how can this kind of system be optimized?
Business magnate Richard Branson claims that "the right people focusing on the right things" can solve almost any problem.
Getting the right people on the right task isn't always so easy. Consider one of the most complex services: medical care. If a patient complaining of certain symptoms first sees a nurse, when — and under what conditions — should a doctor be called in? Assigning patients to staff with the right skillsets at the right time is crucial for both efficiency and positive outcomes. (As is staffing the medical center with the right mix of nurses, residents, fellows, junior and senior physicians.)
A particular problem here is that the nature of each task (illness or problem) is not known in advance; someone has to spend time trying to diagnose a problem before it can be treated. In fact, in this environment, diagnosis is part of the service itself. This creates an operational challenge for service organizations: how can they assign a task effectively, before anyone has learned what it is?
A study by Stanford's Kostas Bimpikis and IESE's Mihalis Markakis addresses this uncertainty in order to improve the performance and efficiency of companies providing services, by reducing misallocated resources. They work with mathematical models to deliver two insights: (1) what kind of data is needed to design and run service systems optimally when learning about the task is part of the service and (2) why the traditional triage (hierarchical) system works so well in health care — as well as in call centers and crowdsourcing systems, as the authors note.
Designing for uncertainty
According to data, the worst performances happen when service providers require a lot of time to learn what kind of task they are dealing with. Another problem area is when tasks don't follow "standard" timelines for resolution and require customization. Such uncertainties affect a company's ability to deal with task flow effectively, and management may be forced to employ more senior staff, elevating staffing costs.
Bimpikis and Markakis find that, in order to optimize a service system where diagnosis is part of the service, management should gather fine-grained data on service times. This data should include the distributions of interarrival times — i.e., the time between the arrival of each client — and the service times themselves — including how much of that total service time is spent on learning about, setting up and delivering the service. For example, service time distributions show what percentage of cases are "solved" after the first 10 seconds of the consultation, what percentage after 20 seconds, and so on. Mapping out this data in a graph, management can see if their distributions have "heavier tails" — i.e., a higher level of unpredictable variability — which would imply greater performance loss due to task-type uncertainty.
According to the authors' mathematical models, optimal system design will have all patients see more junior staff first, until a certain point in time (which is to be appropriately determined), after which they are bumped up to a more senior level to treated more efficiently. This hierarchical or pyramid-like structure helps protect the most senior staff members from being overburdened while serving more patients in a timely manner. This structure is efficient even when the junior staff fails to make a diagnosis in the allotted time, bumping up that part of the task to the more senior staff member.
The authors show that their findings are robust in a variety of cases: (1) when the skill levels of service staff members are optimally decided by the firm, e.g., via training; (2) when the service constitutes of a sequence of tests, such as problem/disease diagnosis; (3) when, during a handover between a junior and senior staff member, at least part of the service that is already provided is "transferable."
Understanding the uncertainty that comes with constantly facing new tasks is key to efficient performance in many service companies. Taking this research into account, managers can turn to models to make better decisions on staffing, training and resource allocation — and run more effective organizations.
Methodology, very briefly
The authors design mathematical models for various service-system scenarios, first using a benchmark model that divided tasks into Easy and Hard types and service professionals into Junior and Senior staff. They modelled different possibilities across organizations' staff, task type, service time, and resource allocation policies, and subsequently extended their analysis to a more general service-system model with greater variability in staff's ability to train and move from Junior to Senior categories, among other factors.