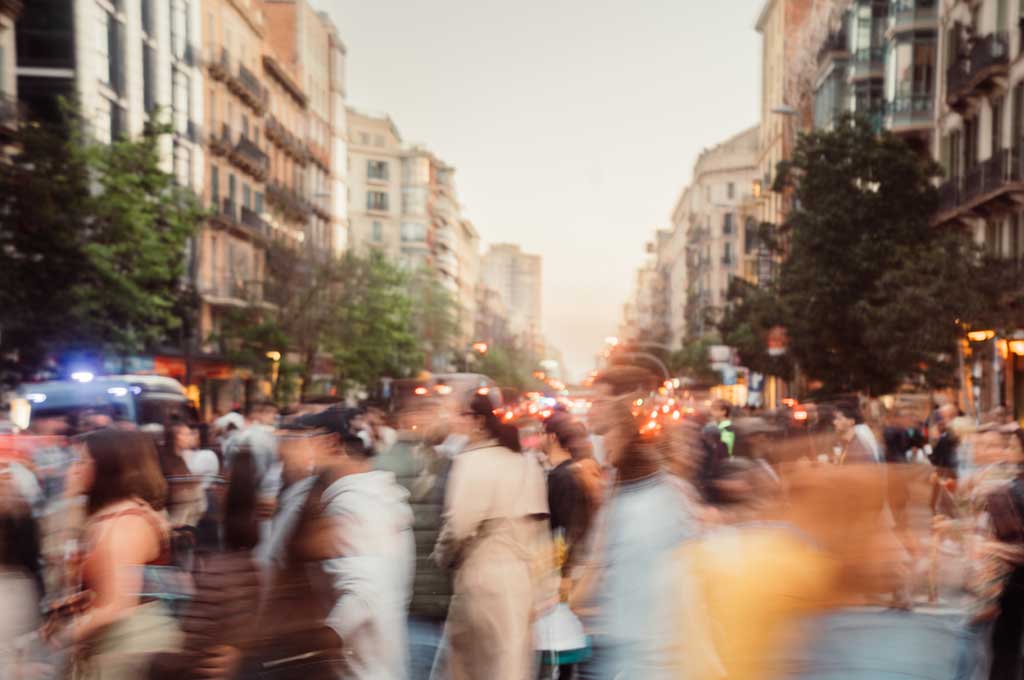
IESE Insight
Betting (and winning) on the wisdom of crowds
How one trader beat the polls and made millions by predicting Donald Trump’s election win.
When French trader “Theo” placed a multimillion-dollar bet on Polymarket ahead of the U.S. presidential election, predicting a win for Trump, accusations of market manipulation quickly surfaced.
Critics suggested that the highly visible trade on the blockchain-based prediction market was an attempt by a pro-Trump agent to create the illusion that Trump was more likely to win. The story gained traction, fueling speculation about an orchestrated effort to sway public perception. Theo has denied these allegations, insisting he just wanted to make money.
In any case, the more intriguing question is: how did Theo manage to predict the election outcome more accurately than the polls?
Asking the right questions
IESE professor Manuel Mueller-Frank believes he knows exactly what gave this trader the confidence to make this huge trade, which resulted in an estimated $85 million profit. In comments to The Wall Street Journal, Theo explains that he based his bets on what is called the “neighbor method,” which shares some conceptual features with an aggregation mechanism introduced by Mueller-Frank and his co-authors Yi-Chun Chen and Mallesh M. Pai in their paper “The wisdom of the crowd and higher-order beliefs.”
It turns out, traditional polling might be asking the wrong question. Instead of directly asking voters, “Who will you vote for?” — a question that may provoke dishonesty, especially in a contentious election — the authors suggest an alternative approach. Ask:
- Who do you believe will win?
- Who do you believe others think will win?
Rather than probing personal voting intentions, these questions focus on respondents’ belief about the aggregate outcome and so-called “second-order beliefs” — what others believe the outcome will be. This conceptual approach is a refined and extended take on an idea that was first implemented by MIT professor Drazen Prelec and co-authors in a mechanism called “Surprisingly Popular.”
It offers several advantages. First, people tend to be more honest when asked about what they think will happen, rather than their own voting intentions. Second, asking additionally about second-order beliefs solves a problem that is present even when respondents are honest about their preferences. That is, generally first-order reports are not enough to identify the true outcome.
By asking these two simple questions about aggregate outcomes — eliciting first-order responses (personal beliefs) and second-order responses (beliefs about others’ beliefs) — the “wisdom of the crowd” aggregates the true outcome with high probability, vastly outperforming expert and pundit predictions.
“Surprisingly Popular is a beautiful procedure, but it has limited applicability,” says Mueller-Frank. “The downside is that it only works in cases of binary outcomes. It was also understood to work only for specific information structures.”
The question Mueller-Frank and his co-authors considered was how to construct a general procedure that worked for multiple potential outcomes and general information structures.
A general and robust mechanism
Building on the conceptual framework of first- and second-order responses, the authors developed a mechanism that transcends the limitations of binary outcomes. Rather than being confined to a simple Trump vs. Harris scenario, their method works for environments with any number of candidates or outcomes.
At its core is “population mean-based aggregation,” a mathematical function that maps individual reports into an aggregated prediction. This approach builds on Prelec’s foundational ideas but diverges significantly in execution.
Instead of asking, “Who will win?” they refine Prelec’s approach by asking for more detailed probabilities and then apply a different mathematical aggregation procedure. The questions they ask are:
- First-order: “What is the probability Trump will win?”
- Second-order: “What do you think is the average reported first-order probability of Trump winning?”
By gathering responses from a sufficiently large number of participants, the mechanism effectively aggregates beliefs into highly accurate predictions. This versatile method demonstrates the power of crowd wisdom, whether in forecasting election results, identifying misinformation or predicting the next World Cup champion.
The polls don’t work
Quite simply, polling methods haven’t worked reliably for some time. Traditional polling methods are ripe for disruption across various fields, most notably in sports betting and election polling. There are myriad examples of the failure of outdated approaches to predict contentious races, with the surprise outcomes of the 2016 U.S. presidential election, the Brexit referendum and the 2014 Scottish independence vote highlighting repeated polling errors.
For any question of aggregation, “second-order elicitation” can be a game-changer, providing a robust method for aggregating outcomes. If widely implemented, this approach could result in a more accurate public understanding of the probabilities of major events. This could also lead to more informationally efficient prices in bond markets, stock markets and prediction markets.
Another advantage is that it is incentive-compatible — though this isn’t foolproof and can be vulnerable to manipulation. The system works well when participants are motivated purely by monetary rewards, encouraging them to share their true beliefs. It falls short if participants care more about influencing the aggregated outcome than about their financial incentives.
Nevertheless, this research lays a strong foundation for making more accurate predictions for general environments and is robust to respondents’ limited understanding of the environment.
Crucially, it doesn’t rely on participants being perfectly rational, making it highly adaptable to real-world scenarios. It also has potential to transform areas like decision-making, forecasting and combating misinformation.