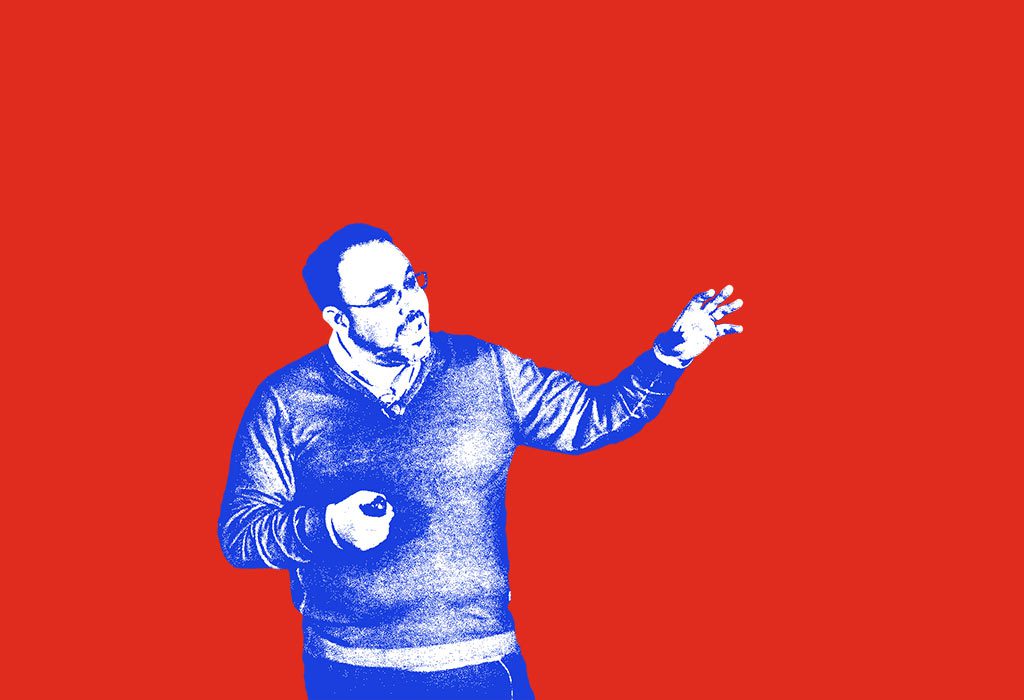
IESE Insight
Artificial intelligence: between promise and adoption
IESE’s Sampsa Samila in conversation with Joshua Gans
For AI to transform entire systems, it takes time and requires new mindsets. System change will come. Here’s what to watch for.
For many of us, 2023 was the year that generative artificial intelligence exploded into our workplaces, classrooms and homes. But for Joshua Gans, 2023 was the year that AI was poised in between, putting its vast potential on display but not yet delivering the system-wide innovation that will upend entire industries.
Gans is a professor at the University of Toronto’s Rotman School of Management and chief economist of the Creative Destruction Lab, a seed-stage program for deep-tech startups. Together with Ajay Agrawal and Avi Goldfarb, Gans is the co-author of Power and prediction: the disruptive economics of artificial intelligence. He came to IESE Business School in November 2023 to speak at an event organized by IESE’s Artificial Intelligence and the Future of Management Initiative, led by professor Sampsa Samila.
Here, they discuss how AI can slash costs, supercharge prediction and transform industries the way electricity did.
Sampsa Samila: As an economist, you often delve into discussions about cost structures and economic efficiency. Could you elaborate on the significance of thinking about the cost of different activities for understanding artificial intelligence?
Joshua Gans: When you look at artificial intelligence, the question you want to ask yourself is: What are these new technologies reducing the cost of? When I say new technologies, I’m talking about machine learning, neural networks, deep learning and now generative AI. What is all this artificial intelligence reducing the cost of?
The answer we found (which turned out to be enlightening but not terribly controversial) was prediction. What artificial intelligence is doing is reducing the cost of prediction — that is, the process by which you use the information you have in order to generate the information you don’t have.
AI translation, just another form of prediction
SS: Given the pervasive influence of AI in both business and societal discourses recently, would categorizing it merely as a cost-reduction tool for prediction oversimplify its multifaceted impact and potential?
JG: Only when you imagine using AI simply to predict things that we are already familiar with, such as weather forecasting or fraud detection. But there is more to prediction than just predicting the future, as in the examples I just mentioned. There is a lot of prediction involved in obtaining the information we need to make decisions. In this sense, the primary benefit of AI prediction is to enhance decision-making.
AI as prediction is also now being applied to things we didn’t previously think of as prediction. For instance, we used to think of translation as the work of someone skilled in two languages or the linguistic rules that converted text from one language to another. But AI translation is taking a piece of English text and predicting how a Spanish speaker, for example, would say the same thing. It’s another prediction problem that gets trained in the same way as we trained weather predictions, although we may not have considered translation as prediction like that before.
Self-driving cars and other limitations of AI
SS: Given the impressive advancements in AI, particularly in domains like translation and textual composition, could we also identify specific areas where AI still demonstrates notable limitations or shortcomings?
JG: A few years ago, there was a lot of optimism that by 2023 we would have self-driving cars. This is something people have believed AI could do for a long time because cars are not that complicated an instrument. But what’s complicated is the environment around the cars and knowing what to do in that environment.
Therein lies the importance of remembering that the technology is fundamentally a statistical process that must abide by the laws of statistics. While in some situations, you can get very, very good predictions when it comes to driving in the human world, it turns out there are errors involved in it, because nothing is ever perfect. So, AI driving has never been able to get perfect enough for us.
Of course, the counterargument is that humans aren’t perfect, either — that we are, in fact, also terrible on that same metric. But we have one other thing that AI doesn’t have, which is, when we make a mistake, we can realize it and correct it. Training AI to realize its mistakes and respond to them is something that hasn’t been done yet. Can it be done? Possibly.
What is new about generative AI?
SS: Apart from artificial intelligence in general, let’s talk more specifically about generative AI. Does generative AI, with all its capacity to create texts, music and images, fit into this prediction paradigm or does it go beyond it?
JG: Generative AI is actually another example of prediction technology. It does exciting things: you can interact with it, it can create stories, it gives you information and it can help you out. Yet essentially — with texts, for example — all it is doing is taking the chunks of words that are your prompt and predicting what the next right chunk of words would be.
SS: So what is new about generative AI?
JG: One thing that’s very new is that it’s widely available at no cost, which, when you think about it, is kind of a miracle.
The second thing is, it can do something that traditionally has been very difficult, which is to generate measurable increases in productivity. Most economists had given up measuring productivity in the service industry because it was so difficult. Now, large language models are so useful at certain tasks that we can measure productivity gains.
Take coding, for instance. We have seen a 50% reduction in the time it takes to code certain tasks — not just simple tasks but really complicated ones as well. We have seen a 37% increase in productivity in generating routine business memos. All of this is now possible and currently measurable, even though we have only just started with this technology.
It’s also important to note that studies have shown that AI benefits low-skilled workers most, because it effectively mimics the abilities of a very highly skilled person.
Why aren’t firms exploiting AI to its full potential?
SS: If that’s true, why have more firms not adopted it?
JG: A lot of firms have actually invested in AI, but they did it without thinking through exactly what they were doing. Few of them reported any benefits.
Just purchasing AI because it gives you something like more insights isn’t enough. What you have to think about is whether it’s useful for something that you need to take an action on.
To think through some of these issues, we distinguish between point solutions, application solutions and system solutions.
SS: Could you expand on each of these three types of solutions?
JG: Point solutions improve on an existing procedure and can be adopted independently, without changing the system in which they are embedded.
An application solution goes beyond that, enabling a new procedure that can be adopted independently — but again, the system in which it is embedded remains essentially unchanged.
These solutions are the forecasting, fraud detection and translation that I mentioned earlier. They aren’t bad and they can really improve productivity. We are seeing more and more of them now.
However, in many cases (and possibly most cases), the benefits of better and cheaper prediction require changes in other, related activities in order to realize the full benefits. Without those, you don’t get transformation — the changes that transform industries until they are unrecognizable. That requires system-level innovation, whose benefits could be quite substantial. But because so many things have to be changed, the adoption of the technology in these cases is inevitably slow.
SS: Where are most businesses on the spectrum of point solutions, application solutions and system solutions?
JG: Right now, we are in what we call “the between times.” We have seen the great promise of this technology, the great ability for point solutions, but the system-level innovations are still not there. Coming up with new systems takes time, because we don’t understand the technology enough, and we also don’t have significant enough improvements to make it worthwhile.
Sometimes people get ahead of themselves. Sometimes people take a technology, such as virtual reality (VR), and say we can push everybody into the metaverse and try to pursue that vision. One of the reasons why that is hard to do is because you might have that vision, but the number of things that have to fall into place and how it gets delivered still have to be worked out. More often than not, the new technology comes in, and we find some great applications for it initially, but it takes us a while to see where the new systems would be.
At this moment, there are no industries that have been reconstituted and completely transformed because of artificial intelligence. There are a lot of people with a lot of ideas of how that would and could occur. It’s safe to say there is a huge amount of uncertainty going on. I don’t know what the new systems will be, and neither do, I’m guessing, most people. But system change will come.
Moving from a task mindset to a system mindset
SS: How can companies move from point solutions to system solutions?
JG: For AI adoption to be successful, you need to have a system mindset as opposed to a task mindset; a focus on value creation as opposed to cost savings based on labor replacement. If prediction and judgment are the two main ingredients of decision-making, with AI, prediction is shifted to a machine and decoupled from judgment. That may change who provides the judgment.
Once you’ve got the right people with the right mindsets, we suggest starting from scratch. First, articulate your company’s mission. Second, reduce the business to the fewest possible decisions required to achieve that mission. Then, specify the prediction and judgments associated with each of the primary decisions, using the help of reliable AI tools. (See the AI systems discovery canvas.)
Despite the disruption that system-level innovation will bring, companies should initiate this process sooner rather than later. AI confers an advantage on first movers because AI learns, if you have the right feedback loops in place.
When will AI have its light-bulb moment?
SS: When you say that system-wide transformations will occur, what sorts of timelines are you envisioning?
JG: Here, we can learn a bit from history. There is a lot of talk about AI being the new electricity, in the sense of it being hugely transformational in the same way that electricity was back in the 1800s. Although Thomas Edison turned on the light bulb in 1879, if you checked in 20 years later, only a small fraction of factories and households in the United States had any electricity. If Bloomberg media had existed in 1900, you probably would have had articles extolling electricity as the next big thing, while most companies still had nothing to show for it. These are the sort of headlines we are seeing today about AI.
The point is, transformation takes time. For electricity, what took time was not the building of power plants or even convincing manufacturers that electricity was cheaper than steam. What took decades was working out where the systems needed to change in order to accommodate the new tech. With electricity, factories could be redesigned into large, flat installations located outside of expensive city rents. Once that had been worked out, and the advantages were abundantly clear, this new thing then spread everywhere. Likewise, watch for such turning points.
READ MORE: Power and prediction: the disruptive economics of artificial intelligence by Ajay Agrawal, Joshua Gans & Avi Goldfarb (Harvard Business Review Press, 2022).
A version of this interview is published in IESE Business School Insight magazine (Jan.-April 2024).